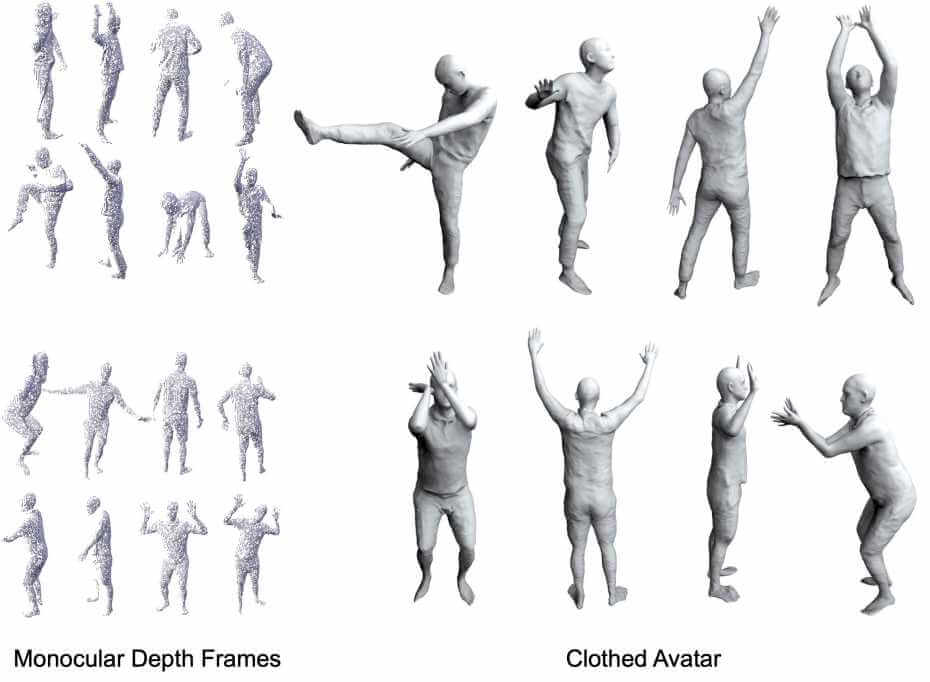
Human avatar creation is required in many applications. Commercial solutions have made tremendous progress in recent years, such as MetaHuman from Unreal. Yet, creating these avatars still needs significant artist efforts. Recent advances in deep learning, mainly neural implicit representations, have enabled controllable human avatar generation from different sensory inputs. However, to generate realistic cloth deformations from novel input poses, watertight meshes or dense full-body scans are usually needed as inputs. Our group has focused on advancing the frontier of realistic human avatar creation from affordable commodity sensors. Our work MetaAvatar is the first approach that created generalizable and animatable neural signed distance fields (SDFs) representing clothed human avatars from monocular RGB sequences. We achieved this by using meta-learning to learn an initialization of a hypernetwork that predicts the parameters of neural SDFs. We demonstrated that our meta-learned clothed human body models are very robust, being the first to generate avatars with realistic dynamic cloth deformations given as few as eight monocular depth frames.
Publications
Authors:Korrawe Karunratanakul, Sergey Prokudin, Otmar Hilliges, Siyu Tang
We present HARP (HAnd Reconstruction and Personalization), a personalized hand avatar creation approach that takes a short monocular RGB video of a human hand as input and reconstructs a faithful hand avatar exhibiting a high-fidelity appearance and geometry.Authors:Marko Mihajlovic, Aayush Bansal, Michael Zollhoefer, Siyu Tang, Shunsuke Saito
KeypointNeRF is a generalizable neural radiance field for virtual avatars.Authors:Shaofei Wang, Katja Schwarz, Andreas Geiger, Siyu Tang
Given sparse multi-view videos, ARAH learns animatable clothed human avatars that have detailed pose-dependent geometry/appearance and generalize to out-of-distribution poses.Authors:Shaofei Wang, Marko Mihajlovic, Qianli Ma, Andreas Geiger, Siyu Tang
MetaAvatar is meta-learned model that represents generalizable and controllable neural signed distance fields (SDFs) for clothed humans. It can be fast fine-tuned to represent unseen subjects given as few as 8 monocular depth images.Authors:Shaofei Wang, Andreas Geiger and Siyu Tang
Registering point clouds of dressed humans to parametric human models is a challenging task in computer vision. We propose novel piecewise transformation fields (PTF), a set of functions that learn 3D translation vectors which facilitates occupancy learning, joint-rotation estimation and mesh registration.