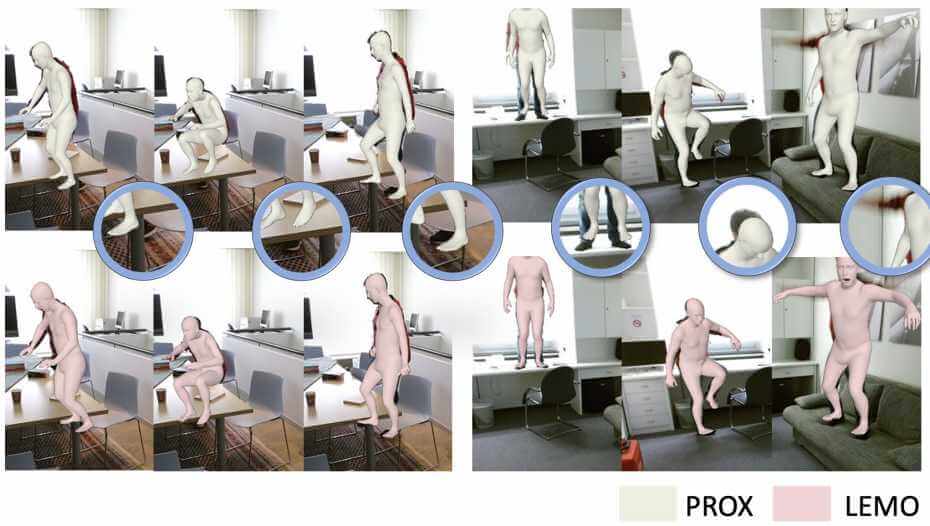
Abstract
Recovering high-quality 3D human motion in complex scenes from monocular videos is important for many applications, ranging from AR/VR to robotics. However, capturing realistic human-scene interactions, while dealing with occlusions and partial views, is challenging; current approaches are still far from achieving compelling results. We address this problem by proposing LEMO: LEarning human MOtion priors for 4D human body capture. By leveraging the large-scale motion capture dataset AMASS, we introduce a novel motion smoothness prior, which strongly reduces the jitters exhibited by poses recovered over a sequence. Furthermore, to handle contacts and occlusions occurring frequently in body-scene interactions, we design a contact friction term and a contact-aware motion infiller obtained via per-instance self-supervised training. To prove the effectiveness of the proposed motion priors, we combine them into a novel pipeline for 4D human body capture in 3D scenes. With our pipeline, we demonstrate high-quality 4D human body capture, reconstructing smooth motions and physically plausible body-scene interactions.