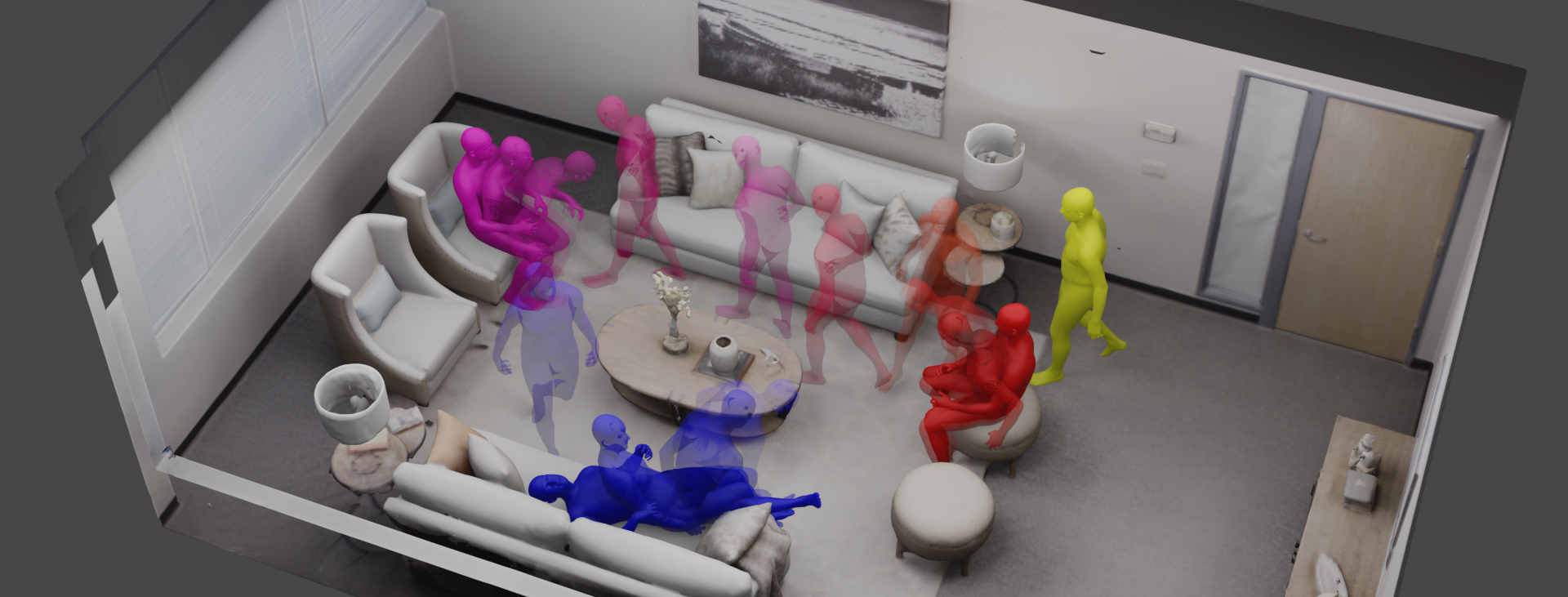
Abstract
Interaction with environments is one core ability of virtual humans and remains a challenging problem. We propose a method capable of generating a sequence of natural interaction events in real cluttered scenes. We formulate synthesizing human behaviors in 3D scenes as a Markov decision process with a latent action space, which is learned from motion capture datasets. We train scene interaction-aware and goal-driven agent policies to synthesize various human behaviors in indoor scenes including wandering in the room, sitting or lying on an object, and sequential combinations of these actions.