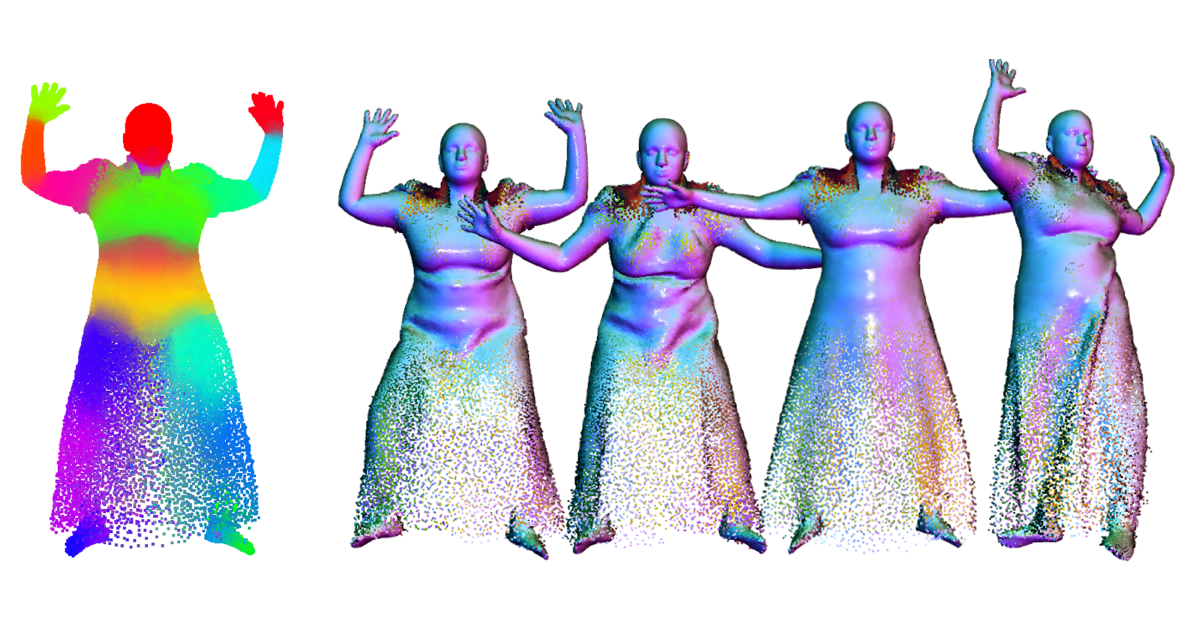
Abstract
Parametric 3D body models like SMPL only represent minimally-clothed people and are hard to extend to clothing because they have a fixed mesh topology and resolution. To address this limitation, recent work uses implicit surfaces or point clouds to model clothed bodies. While not limited by topology, such methods still struggle to model clothing that deviates significantly from the body, such as skirts and dresses. This is because they rely on the body to canonicalize the clothed surface by reposing it to a reference shape. Unfortunately, this process is poorly defined when clothing is far from the body. Additionally, they use linear blend skinning to pose the body and the skinning weights are tied to the underlying body parts. In contrast, we model the clothing deformation in a local coordinate space without canonicalization. We also relax the skinning weights to let multiple body parts influence the surface. Specifically, we extend point-based methods with a coarse stage, that replaces canonicalization with a learned pose-independent “coarse shape” that can capture the rough surface geometry of clothing like skirts. We then refine this using a network that infers the linear blend skinning weights and pose dependent displacements from the coarse representation. The approach works well for garments that both conform to, and deviate from, the body. We demonstrate the usefulness of our approach by learning person-specific avatars from examples and then show how they can be animated in new poses and motions. We also show that the method can learn directly from raw scans with missing data, greatly simplifying the process of creating realistic avatars. Code is available for research purposes.